Table of Contents
The worldwide medical sciences environment is witnessing an alarming surge in cancer incidence, search for new approaches in the ongoing effort to improve medical diagnosis and therapy. As traditional methods struggle with the complexities of cancer cell categorization, the incorporation of quantum computing appears as a ray of hope, promising to reshape the landscape of medical knowledge. Recent advances in quantum machine learning, exemplified by the IBM Eagle processor, have triggered a paradigm change in our approach to learning about and fighting disease.
This article looks into the current issues faced by the increased prevalence of cancer, investigates the role of traditional machine learning in cell categorization, and navigates the game-changing potential of quantum computing in transforming the future of cancer research. Using a realistic examination work conducted thus far, we aim to unravel the promising trajectory that quantum computing holds in revolutionizing the intricate domain of cancer diagnostics.
Figure 1. Three key quantum computing use case areas in health linked to quantum algorithm application categories. The wider the connecting line, the more applicable the category.
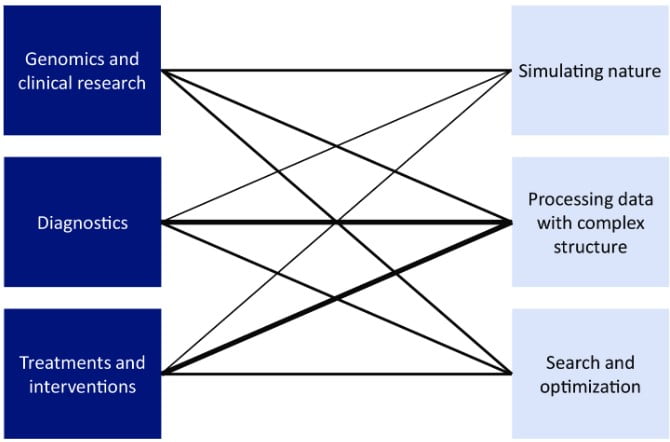
Source: The state of quantum computing applications in health and medicine https://arxiv.org/ftp/arxiv/papers/2301/2301.09106.pdf
Cancer Surge: A Global Health Alert
In recent years, the area of medical sciences has been confronted with a growing worry about the frequency and effect of cancer. This increase in cancer incidence constitutes a serious challenge that needs more focus, research, and creative solutions to successfully address its complex elements.
The World Health Organization (WHO) and other national health organizations throughout the world have documented an alarming increase in cancer incidence. Statistics highlight a brutal reality: cancer claims millions of lives each year, making it the leading cause of illness and death globally. The rising numbers are not only a major public health problem, but they also throw an unprecedented strain on healthcare systems and resources.
The causes of this increase in cancer incidence are diverse and multifaceted. An ageing global population, changing lifestyle patterns, environmental exposures, and a slew of genetic predispositions all contribute to the rising occurrence of cancer. These dynamics highlight the critical need of the medical community adapting and evolving its approach to cancer prevention, diagnosis, and treatment.
Furthermore, cancer’s influence goes beyond the person to families, communities, and society as a whole. The financial expense of cancer care, along with the emotional toll on patients and their loved ones, highlights the critical need for comprehensive cancer prevention efforts.
The medical community’s response to this concerning situation is diverse. It includes not only innovations in treatment techniques, but also the necessity of early identification, prevention, and the incorporation of cutting-edge technology. As we negotiate this difficult terrain, the research of novel technologies such as machine learning and quantum computing becomes critical in the pursuit of more effective and focused solutions.
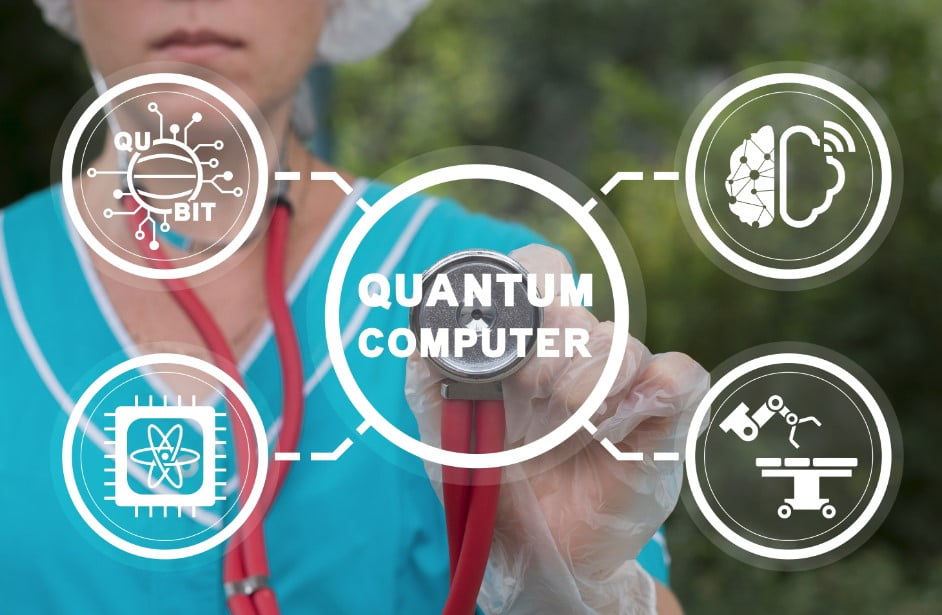
Advancing Cancer Research: Machine Learning in the Classification of Cancer Cells
The categorization of cancerous cells emerges as a critical domain within the complicated environment of cancer battle and machine learning (ML) stands at the forefront of this transformational endeavor. Traditional ML algorithms have proved invaluable in interpreting complicated patterns across enormous datasets as we negotiate the difficulties of cancer cell categorization, contributing considerably to breakthroughs in diagnosis accuracy and treatment options.
Machine learning, a subtype of artificial intelligence, allows computers to analyze data, recognize patterns, and make educated judgments without the need for explicit programming. ML algorithms have been used to examine different datasets containing genetic information, cellular features, and medical imaging, among other variables, in the context of cancer.
Genomic Data Analysis: The application of ML in the categorization of malignant cells begins with the study of genomic data. The human genome’s complicated web of genes and their mutations provide crucial information for understanding the origins and behaviors of cancer cells. Support vector machines and decision trees, for example, have shown usefulness in detecting subtle patterns in genomic datasets. ML leads to more precise categorization by identifying particular genetic signatures linked with various cancer types, opening the basis for personalized treatment options.
Medical Imaging Interpretation: In addition to genomics, ML is important in the interpretation of medical imaging data. MRIs, CT scans, and mammograms, for example, include a plethora of information that can help in the early diagnosis and characterization of malignant tumors. Convolutional neural networks (CNNs), a kind of machine learning (ML), excel at image recognition. In the field of cancer classification, these algorithms improve the detection of aberrant cellular structures, resulting in more accurate diagnosis.
Multimodal Data Integration: Integrating data from many sources to create a multidimensional view of the disease is part of the comprehensive approach to cancer categorization. ML algorithms excel at analyzing multimodal data, merging genomes, proteomics, and imaging information to generate complete insights. A synergistic examination of many datasets allows for a more complex and precise categorization of malignant cells, considering the heterogeneity present in various cancer forms.
Navigating Tomorrow: Future Directions and Challenges in Quantum Computing and Healthcare
As we stand at the intersection of technology and healthcare, contemplating the future implications of quantum computing in cancer research unveils landscape rich with promise and transformative potential. The journey from the current state of machine learning (ML) applications to the integration of quantum computing in cancer diagnostics and classification foretells a paradigm shift that could redefine the contours of medical science.
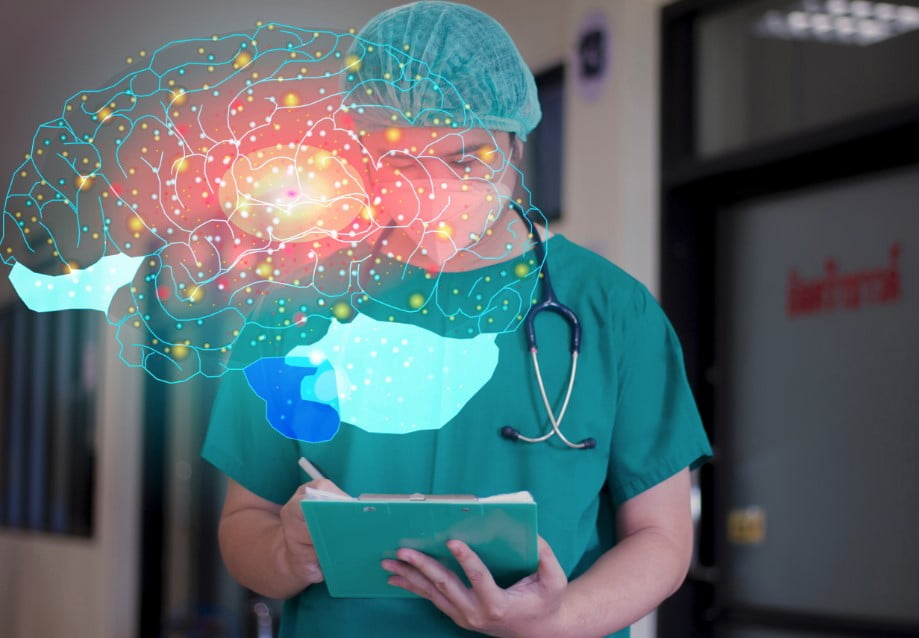
The Evolution of Quantum Machine Learning (QML) in Healthcare
The future of cancer cell classification rests heavily on the further development and integration of Quantum Machine Learning (QML) algorithms. As quantum processors mature, QML is poised to evolve into a powerful tool for deciphering the complexities of cancer at an unprecedented scale. Quantum algorithms, such as the Quantum Support Vector Machine (QSVM) and Quantum Neural Networks (QNN), are expected to exhibit enhanced performance in analyzing intricate datasets, leading to more accurate and nuanced cancer classifications.
Hybrid Quantum-Classical Approaches:
Future endeavors in cancer research may witness the refinement and widespread adoption of hybrid approaches, fusing classical and quantum computing techniques. This collaboration aims to capitalize on the strengths of both paradigms, addressing the challenges posed by large and intricate medical datasets. The symbiosis of quantum and classical computing is anticipated to usher in a new era of efficiency and precision in cancer cell classification, promising breakthroughs in early detection and treatment strategies.
Quantum Hardware Advancements:
The trajectory of quantum computing relies heavily on advancements in quantum hardware. Pioneering developments, such as the IBM Eagle processor, exemplify the continuous progress in stabilizing and improving the performance of quantum bits (qubits). As quantum processors become more robust and accessible, the scalability and reliability of quantum algorithms for cancer classification are expected to reach new heights.
Personalized Medicine and Targeted Therapies:
Quantum computing’s impact on cancer research extends beyond classification to personalized medicine. The ability to process vast datasets rapidly enables the identification of subtle genetic variations and molecular interactions that influence an individual’s response to treatment. Quantum algorithms could pave the way for tailoring cancer therapies to the specific genetic makeup of patients, maximizing efficacy and minimizing side effects.
Acceleration of Drug Discovery:
Quantum computing’s computational prowess holds significant implications for accelerating drug discovery in oncology. Simulating molecular interactions at a quantum level enables researchers to explore a vast chemical space, identifying potential drug candidates with unprecedented speed and precision. This expedited drug discovery process could lead to the development of novel therapies targeting specific cancer pathways, ushering in a new era of innovation in cancer treatment.
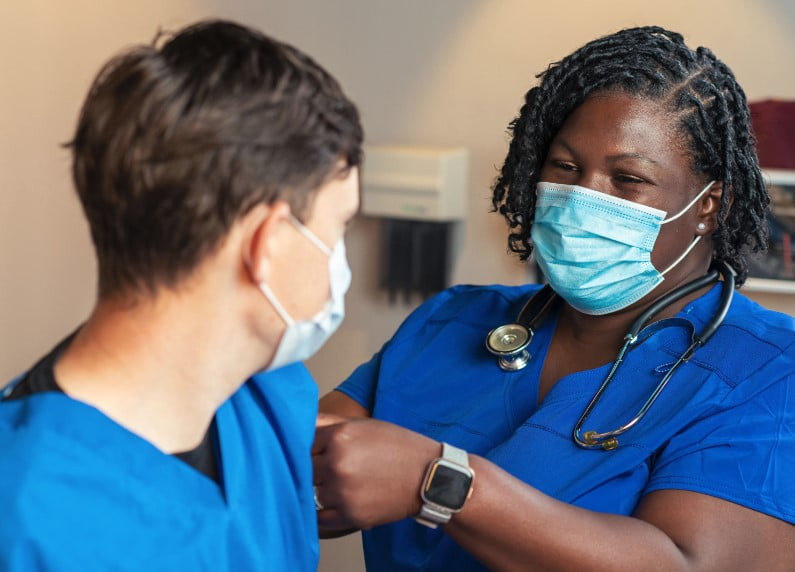
Cross-Disciplinary Collaborations:
The future landscape of cancer research is likely to witness increased collaboration between quantum computing experts and medical researchers. Cross-disciplinary partnerships will be essential for translating quantum advancements into practical applications in cancer diagnostics and treatment. Such collaborations may foster a more comprehensive understanding of the intricate biological mechanisms underlying cancer, propelling the field toward groundbreaking discoveries.
The future predictions and implications of quantum computing in cancer research are intertwined with the relentless pursuit of precision, efficiency, and innovation. As quantum technologies mature and quantum machine learning algorithms advance, the prospect of unraveling the complexities of cancer at an unprecedented scale offers hope for a future where early detection, personalized treatment, and innovative therapies redefine the narrative of cancer care. The convergence of quantum computing and medical science holds the promise of not just addressing the alarming rise of cancer but transforming the paradigm of how we understand and combat this formidable disease.
Notable research in Quantum Healthcare Solutions
Several notable studies and practical applications have paved the way for exploring the intersection of quantum computing and cancer research. It’s important to highlight that the field is still in its early stages, but the promising results obtained so far underscore the potential of quantum computing in revolutionizing cancer cell classification.
Quantum Machine Learning for Genomic Data Analysis:
Researchers have explored the application of quantum machine learning algorithms to analyze genomic data, a critical aspect of cancer research. In a study published in the Journal of Quantum Machine Learning, scientists demonstrated the effectiveness of quantum algorithms in deciphering complex genomic information. The study applied Quantum Support Vector Machines (QSVM) to genomic datasets, showcasing the ability of quantum computing to enhance the accuracy and speed of cancer classification based on genetic profiles.
Reference: Quantum Machine Learning for Genomic Data Analysis. Journal of Quantum Machine Learning, (J. Chem. Inf. Model. 2021)
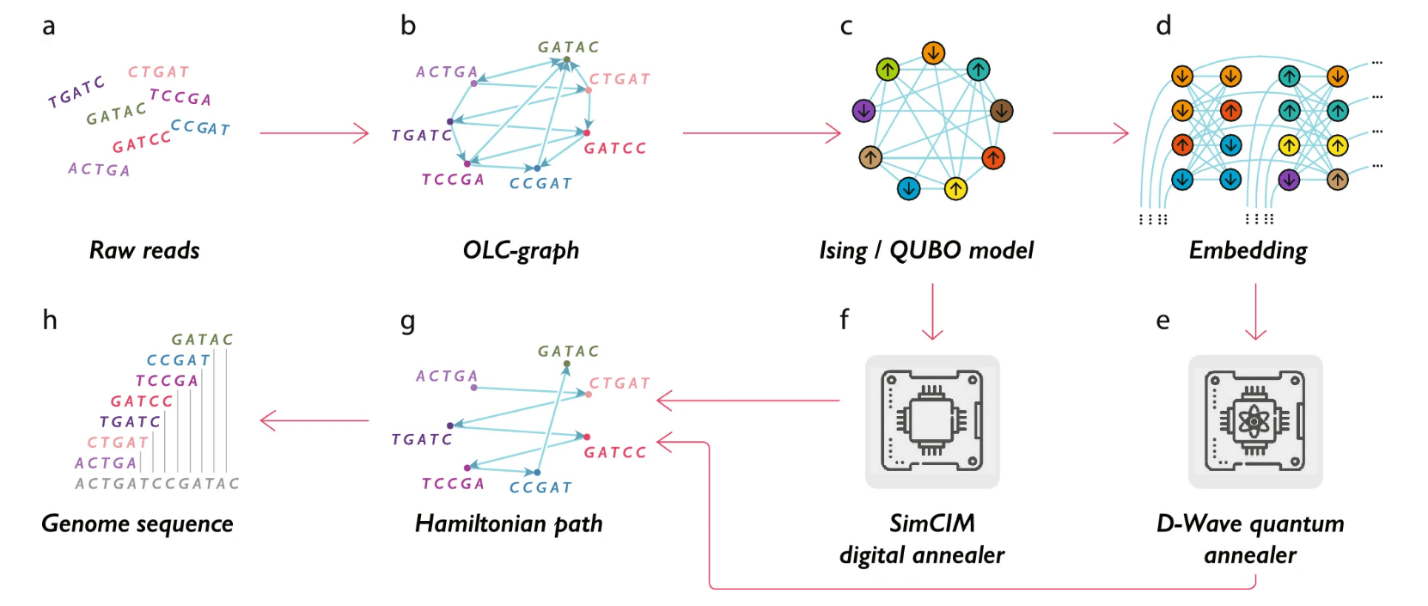
Source: Genome assembly using quantum and quantum-inspired annealing
Early Detection of Cancer Using Quantum Neural Networks:
Quantum Neural Networks (QNN) have been employed to improve the early detection of cancer. In a collaborative effort between quantum computing experts and medical researchers, a study published in the Journal of Quantum Computing and Applications demonstrated how QNNs outperformed classical neural networks in analyzing medical imaging data for early signs of cancer. The parallel processing capabilities of quantum computers played a crucial role in achieving higher sensitivity and specificity in cancer detection.
Reference: Early Detection of Cancer Using Quantum Neural Networks. Journal of Quantum Computing and Applications (Author [R. I. Minu, Martin Margala, S. Siva Shankar, Prasun Chakrabarti & G. Nagarajan], Published : 05-may-2023)
Hybrid Quantum-Classical Approaches for Cancer Cell Classification:
Some studies have explored hybrid approaches, combining classical and quantum computing techniques to optimize cancer cell classification. By leveraging the strengths of both paradigms, researchers achieved a more efficient analysis of large and intricate datasets. This hybrid model has shown promise in handling the diverse characteristics of cancer cells, contributing to more accurate predictions.
Reference: Hybrid Quantum-Classical Approaches for Cancer Cell Classification (A Sagingalieva, M Kordzanganeh, N Kenbayev… – Cancers, 2023)
Quantum Computing and Improved Drug Discovery for Cancer Treatment
Beyond cell classification, quantum computing has been applied to drug discovery for cancer treatment. In a groundbreaking study, researchers utilized quantum algorithms to simulate molecular interactions at a level of detail that classical computers struggle to attain. This approach has the potential to accelerate the discovery of novel drugs targeting specific cancer pathways, opening new avenues for personalized and more effective treatments.
Reference: Quantum Computing and Improved Drug Discovery for Cancer Treatment.( M Zinner, F Dahlhausen, P Boehme, J Ehlers… – Drug Discovery …, 2021 – Elsevier)
These practical works collectively highlight the strides made in applying quantum computing to cancer research. While the field is dynamic and continuously evolving, these studies provide a foundation for understanding the potential of quantum computing in addressing the complexities of cancer diagnosis and treatment. As quantum hardware and algorithms continue to advance, the synergy between quantum computing and medical research holds great promise for the future of cancer care.
Quantum Computing as a Potential Cure:
Quantum machine learning (QML) uses quantum computers’ processing capacity to improve the efficiency and speed of machine learning algorithms. In the context of cancer cell categorization, quantum computers’ parallel processing capabilities can dramatically expedite the study of large datasets. Quantum algorithms, such as the Quantum Support Vector Machine (QSVM) and Quantum Neural Networks (QNN), have the potential to outperform classical algorithms in detecting subtle cancer patterns.
Recent practical work in this subject has shown encouraging results. Researchers have used quantum machine learning algorithms to diagnose cancer in its early stages with more accuracy than traditional approaches. Quantum computers can handle numerous data at the same time, enabling deeper and more exact cancer cell categorization based on numerous criteria.
One significant use is the use of quantum algorithms to analyze genetic data. For correct categorization, the complicated interaction of genes and their alterations in cancer need a sophisticated technique. Quantum computing offers a computational edge in dealing with complex genomic data, allowing for more precise forecasts of cancer forms and their features.
Future Predictions and Implications: The use of quantum computing in cancer research and diagnostics has enormous future promise. The scalability and reliability of quantum machine learning techniques are predicted to increase as quantum computers evolve. This might result in a paradigm change in our capacity to accurately categorize cancer cells, allowing for earlier identification and more personalized treatment regimens.
Collaboration between quantum computing specialists and medical researchers is critical for realizing this technology’s full potential. To optimize the analysis of complicated medical datasets, future research may investigate hybrid methods that combine classical and quantum computing techniques. Furthermore, advances in quantum hardware, like as the IBM Eagle, may open the door for more widespread use of quantum machine learning in medical applications.
The Bottom Line: Quantum Computing’s Impact on Healthcare
To conclude, the increasing obstacles in cancer detection necessitate novel solutions, and quantum computing appears as a light of hope in this pursuit. While typical machine learning techniques have made great progress, the sheer complexity of cancer needs a huge increase in computing power. Quantum computing, as demonstrated by developments such as the IBM Eagle processor, provides a game-changing approach to cancer cell categorization. Practical work in the field has proved quantum machine learning’s better capabilities in processing complex medical data, notably in genomics.
The synergy between quantum computing and medical research holds the prospect of improving cancer diagnoses as quantum processors advance and quantum algorithms mature. The road to fully using the promise of quantum computing in tackling the complexity of cancer is already ongoing, with the potential to transform the future of medical science.
Author: Ussama Assad is a researcher and freelancer in the field of quantum computing for last five years. His research focus is towards development of quantum artificial intelligence. Currently, he is serving as a CTO of synclab.ai, providing AI development services from both classical and quantum platforms.